News cloud representing the semantic closeness or remoteness between news items - EUROPA PRESS
MADRID, 20 Sep (EUROPA PRESS) -
Can artificial intelligence help journalists detect and combat the rise of fake news? This is precisely the aim of the new R&D project that Europa Press is developing with AyGLOO. This project has the financial support of the Centre for the Development of Industrial Technology (CDTI) and it aims to create tools which will analyse and rapidly detect fake news, in addition to explaining the reasons why they are considered fake.
What problems do we face?
A prediction made by the consultancy Garnet in 2017 put the spotlight on 2022 and claimed that by then, the Western public would consume more fake news than real news. There is no way of checking whether we have already crossed this threshold, but concerns about the rise of fake news have been at the centre of public debate.
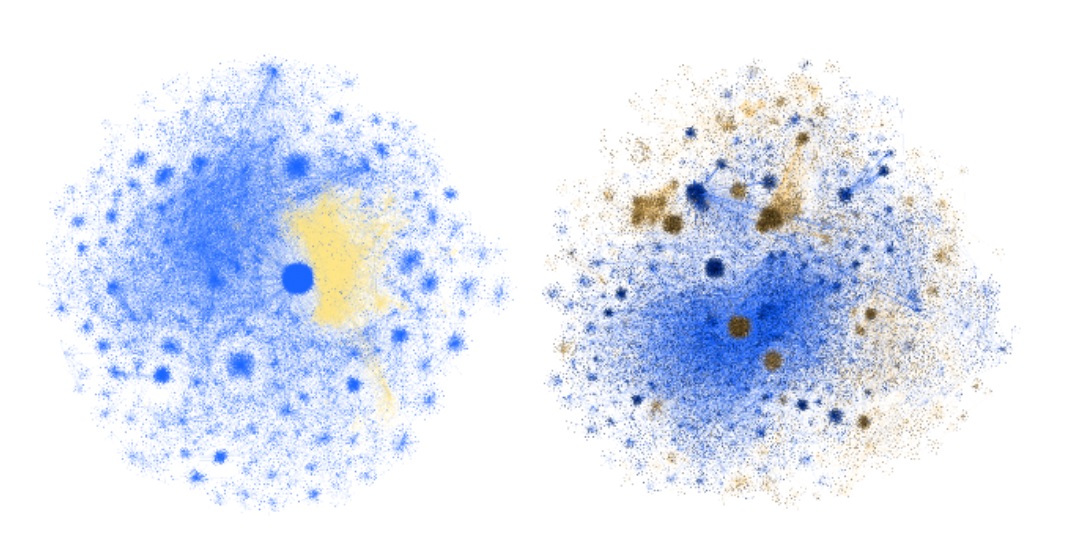
A study by Indiana University shows how fake news (in yellow/brown) spreads through Twitter contacts.
According to the Ministry of Foreign Affairs, European Union and Cooperation, hoaxes and disinformation represent "a global threat to freedom and democracy" that has been aggravated by social networks, and "in recent years both the flow of information and disinformation has accelerated", as demonstrated by the COVID pandemic. A reality that is worsened by studies indicating that eight out of ten Spaniards have difficulty distinguishing between fake and real news.
Who are we?
The European Commission has pointed out that in the fight against disinformation, the coordination of three actors is key: technology companies, civil society (including factcheckers), and academic institutions.
Bringing together the experience of a media company in the information sector, and the knowledge of experts in Artificial Intelligence, is precisely the core of the new Europa Press project, which includes the Europa Press group of developers, the AyGLOO team of Data Scientists, systems architects and programmers, and the collaboration of the Polytechnic University of Madrid, and GIATEC.
Where do we want to go?
The ultimate goal is to provide the editors of Europa Press and other interested media with tools capable of speeding up the news verification process. How? By applying advanced artificial intelligence techniques capable of analysing the text of the news item, and alerting the editor, either that the news item has already been verified by another independent body, or, when necessary, that it is suspected of being fake and should be verified by an expert. An early warning system that could be used as a first 'test' for texts published or shared on social networks, forums, or other areas of the Internet.
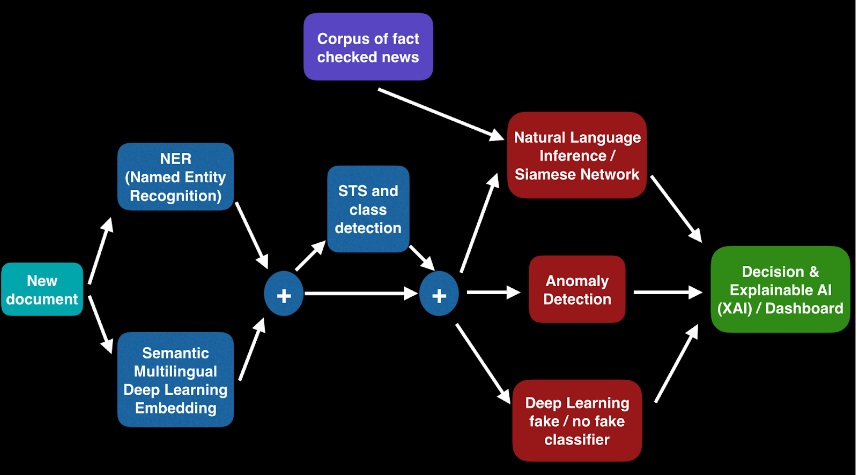
Diagram showing the steps that the fake news early warning tool will follow.
Smart Fake News Detection (FND), as the tool is called, will be characterised by chaining several different artificial intelligence techniques such as NLP (Natural Language Processing), STS (Semantic Text Similarity), NLI (Natural Language Inference), Anomaly Detection, and XAI (Explainable Artificial Intelligence) to the text of the news object being analysed. The output of the tool will finally be presented in a validation, classification, and explanation dashboard.
In order to detect whether a news item is fake or not, each news text will go through a sequence of deep learning artificial intelligence processes that treat the text in a cascade of steps (categorisation and labelling, semantic vectorisation/embedding, similarity search, inference, classification), that will finally determine its veracity.
All these treatments follow a parallel process of explainable artificial intelligence (XAI), in order to maintain transparency and confidence in the process followed by our FND tool.
News cloud representing the semantic closeness or remoteness between news items. Each point is a news item. Each colour represents the type of news identified by the AI algorithm.
Where are we now?
The project began in early 2022 and the first step of the process: natural language analysis (NLP), responsible for categorising the news item and NER (Named-Entity Recognition) analysis, to detect and label entities within the news item, has already been completed.
To do this, starting from a dataset of more than 16,000 news items from Europa Press, a system was trained where first the semantic coding of the document (a vector with 512 components) is extracted by means of a deep neural network of the transformer type, and then this vector is classified into one of the categories by means of an advanced neural network. The categories assigned by the network often do not coincide with those assigned by Europa Press, as there are news items that can be classified into several sections. For example, there are news items that can be labelled as Education or Society, and there are others that can be labelled as Motor or Economy.
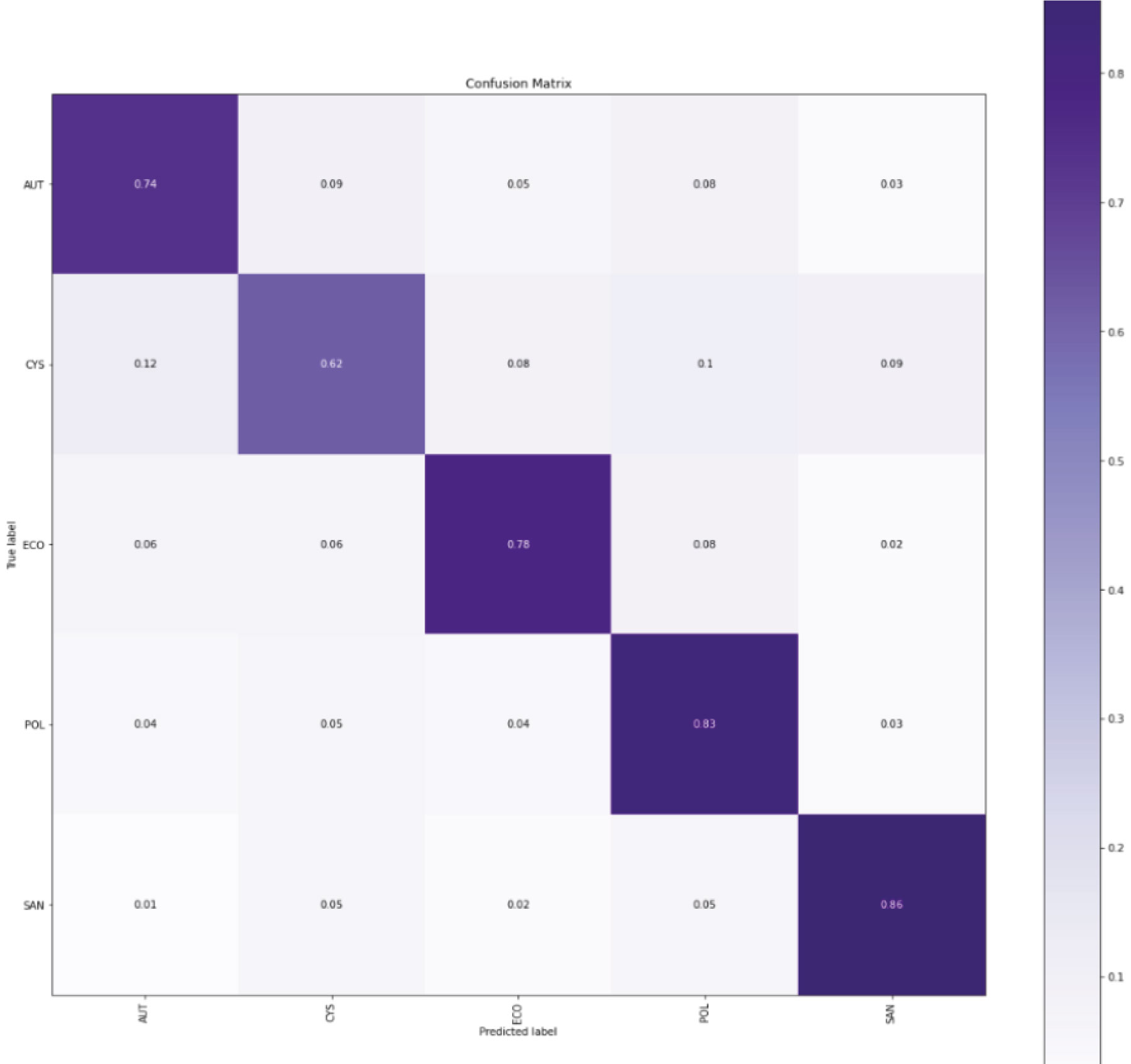
Confusion matrix
"In recent years both the flow of information and disinformation has accelerated"
In a second test, we have only and exclusively included the categories that occur in a set equal or superior to 10% of the total data (AUT, CYS, POL, ECO, SAN) obtaining better results in the classification, and obtaining really good results of true positives and true negatives.
In addition, the AyGLOO team of experts has carried out a comparative analysis of the different NER (Named Entity Recognition) models available to determine which one is the best. This type of model detects entities such as people, places, and organisations, in the text.
As transparency is another of the project's pillars, the team has also made progress on an explainability module for categorising a news text into the most important sections. The sum of the probability that a piece of news can be seen graphically in one section or another.
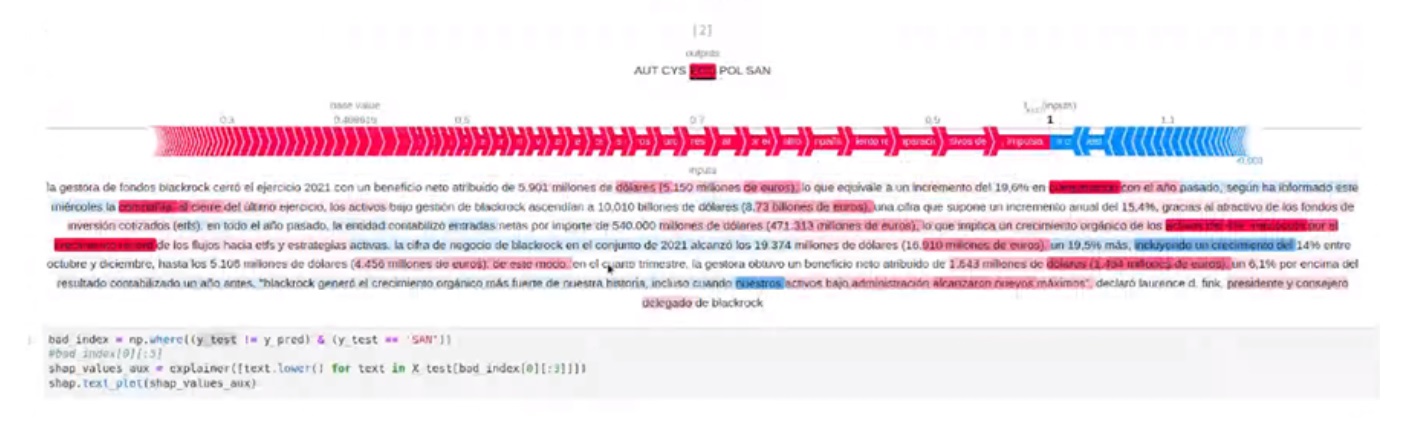
Explainability module developed for multiclass classification
The corpus of verified news fed into the tool includes those claims that have already been verified by independent fact-checking organisations around the world. This is done by querying the Datacommons.org database, which has been adopted by many fake news verifiers and is also used by Google in its search results.
To this end, it has been necessary to implement an ETL process that periodically downloads updated versions of the feed in json format from Datacommons.org's FactCheck tool, which cleans and prepares the information we will use for the searches.
To implement the semantic textual similarity search (STS) engine that our API will use to find verified facts, it is necessary to perform a text embedding process to represent the semantic content of a text written in any language in a numerical vector (dense vector) represented in a multidimensional space. For this purpose, we have used a model for text embedding called Sentence-BERT, which performs sentence embedding using Siamese nets.
Since the indexed documents may belong to more than 50 different languages, it was necessary to present the user with search results in their own language. This included the use of a unique AI model created by Meta AI, the artificial intelligence lab belonging to Meta Platforms Inc, formerly known as Facebook, Inc. That model is the NLLB-200 (No Language Left Behind) which is capable of translating with unprecedented quality into 200 different languages.
Next steps
Among the challenges for the coming months are the development of an inference engine, the Natural Language Inference (NLI) module, to receive and process similar news texts found, to continue with the explainability module, and the search for collaboration with other organisations and companies interested in the fight against fake news.